Practical Fairness: Achieving Fair and Secure Data Models

In the era of big data and artificial intelligence, fairness has become a critical concern in machine learning and data modeling. As data-driven models are increasingly used to make important decisions that affect people's lives, it is essential to ensure that these models are fair and unbiased.
4.8 out of 5
Language | : | English |
File size | : | 7598 KB |
Text-to-Speech | : | Enabled |
Enhanced typesetting | : | Enabled |
Word Wise | : | Enabled |
Print length | : | 347 pages |
Screen Reader | : | Supported |
Paperback | : | 104 pages |
Reading age | : | 9 - 12 years |
Grade level | : | 4 - 6 |
Item Weight | : | 4 ounces |
Dimensions | : | 5 x 0.24 x 8 inches |
What is Fairness in Machine Learning?
Fairness in machine learning refers to the property of a model that makes predictions or decisions without discrimination or bias against individuals or groups of individuals based on protected attributes such as race, gender, or age.
There are different types of fairness that can be considered in machine learning models:
- Individual fairness: This type of fairness ensures that each individual is treated fairly by the model. For example, in a hiring decision model, individual fairness would mean that each candidate is evaluated based on their qualifications and not on any protected attributes.
- Group fairness: This type of fairness ensures that different groups of individuals are treated fairly by the model. For example, in a loan approval model, group fairness would mean that the model does not discriminate against applicants from certain racial or ethnic groups.
- Disparate impact: This type of fairness focuses on the impact of the model on different groups of individuals. For example, in a criminal risk assessment model, disparate impact would mean that the model does not result in disproportionately high false positive rates for certain racial or ethnic groups.
Why is Fairness Important?
Fairness in machine learning is important for several reasons:
- Ethical reasons: It is unethical to use models that discriminate against individuals or groups of individuals.
- Legal reasons: In many countries, there are laws that prohibit discrimination based on protected attributes.
- Business reasons: Models that are not fair can lead to reputational damage and loss of customer trust.
Challenges of Achieving Fairness
Achieving fairness in machine learning models can be challenging for several reasons:
- Bias in data: Data used to train machine learning models can often contain biases that reflect the biases of the society in which the data was collected.
- Algorithmic biases: Machine learning algorithms can themselves introduce biases if they are not designed to be fair.
- Trade-offs between fairness and other objectives: In some cases, achieving fairness may require making trade-offs with other objectives such as accuracy or security.
Practical Fairness
Practical fairness is an approach to achieving fairness in machine learning models that acknowledges the challenges mentioned above and provides guidance on how to mitigate these challenges.
The following are some key principles of practical fairness:
- Start with a fair data set: The first step to achieving fairness is to start with a data set that is as free of bias as possible.
- Use fair algorithms: There are a number of machine learning algorithms that are designed to be fair. These algorithms can help to mitigate the biases that may be present in the data.
- Evaluate fairness: Once a model has been trained, it is important to evaluate its fairness. This can be done using a variety of fairness metrics.
- Make trade-offs carefully: In some cases, achieving fairness may require making trade-offs with other objectives. It is important to weigh these trade-offs carefully and make decisions based on the specific context.
Achieving Fairness in Data Models
The following are some specific steps that can be taken to achieve fairness in data models:
- Identify protected attributes: The first step is to identify the protected attributes that are relevant to the model. This will vary depending on the specific context.
- Audit the data: The next step is to audit the data for biases related to the protected attributes. This can be done using a variety of data auditing techniques.
- Mitigate biases: Once biases have been identified, steps can be taken to mitigate them. This may involve removing biased data, using imputation techniques to correct for missing data, or using algorithms that are designed to be fair.
- Train and evaluate the model: Once the data has been cleaned and the biases have been mitigated, the model can be trained and evaluated. The model should be evaluated for both accuracy and fairness.
- Monitor the model: Once the model has been deployed, it is important to monitor its performance for fairness over time. This will help to ensure that the model remains fair as the data and context change.
Security Considerations
It is important to consider security when implementing practical fairness in data models.
The following are some potential security risks that should be considered:
- Data breaches: Data breaches can expose sensitive data, including protected attributes. This can be used to attack models and undermine their fairness.
- Model manipulation: Models can be manipulated to produce unfair or biased results. This can be done by attackers or by malicious insiders.
- Discrimination: Models that are not fair can be used to discriminate against individuals or groups of individuals. This can have a negative impact on people's lives and livelihoods.
The following are some steps that can be taken to mitigate these risks:
- Protect data: Data should be stored and processed in a secure manner. This includes encrypting data, controlling access to data, and implementing data breach prevention measures.
- Validate models: Models should be validated for both accuracy and fairness. This can be done by using independent data sets and by conducting human audits.
- Monitor models: Models should be monitored for fairness over time. This can be done by using automated monitoring tools or by conducting regular human audits.
Practical fairness is an essential consideration for machine learning practitioners. By following the principles and steps outlined in this article, it is possible to achieve fairness in data models while maintaining security and accuracy.
As the use of machine learning continues to grow, it is more important than ever to ensure that these models are fair and unbiased. By working together, we can create a world where everyone benefits from the power of machine learning.
4.8 out of 5
Language | : | English |
File size | : | 7598 KB |
Text-to-Speech | : | Enabled |
Enhanced typesetting | : | Enabled |
Word Wise | : | Enabled |
Print length | : | 347 pages |
Screen Reader | : | Supported |
Paperback | : | 104 pages |
Reading age | : | 9 - 12 years |
Grade level | : | 4 - 6 |
Item Weight | : | 4 ounces |
Dimensions | : | 5 x 0.24 x 8 inches |
Do you want to contribute by writing guest posts on this blog?
Please contact us and send us a resume of previous articles that you have written.
Book
Chapter
Text
Story
Genre
Library
Paperback
E-book
Paragraph
Sentence
Bibliography
Preface
Synopsis
Annotation
Footnote
Codex
Tome
Classics
Library card
Narrative
Biography
Memoir
Reference
Thesaurus
Resolution
Catalog
Borrowing
Archives
Study
Research
Academic
Journals
Rare Books
Special Collections
Interlibrary
Literacy
Thesis
Storytelling
Awards
Book Club
Sahar Abdulaziz
Matt Jones
Ajay Bhargove
Chris Buono
Frank Watson
Brittany Fichter
Naghilia Desravines
Katharina Bordet
Art Weinstein
Harry Glorikian
Michael Matthews
John Egerton
Martin Adams
Tim Conrad
Malcolm Haddon
Anika Fajardo
Emmanuel Karagiannis
Brandi Davis
Tom Shapiro
Scott Jay Marshall Ii
Light bulbAdvertise smarter! Our strategic ad space ensures maximum exposure. Reserve your spot today!
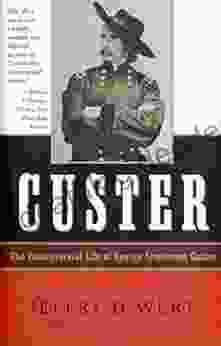

- Demetrius CarterFollow ·5.2k
- Andrew BellFollow ·19.2k
- Forrest ReedFollow ·18.6k
- Gilbert CoxFollow ·10.7k
- Adrian WardFollow ·4.4k
- Jerome PowellFollow ·10.5k
- Bruce SnyderFollow ·7.3k
- Mike HayesFollow ·6.2k
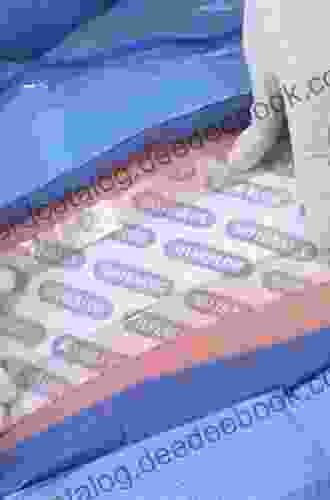

Chronic Wounds, Wound Dressings, and Wound Healing:...
Chronic wounds are a major challenge for...
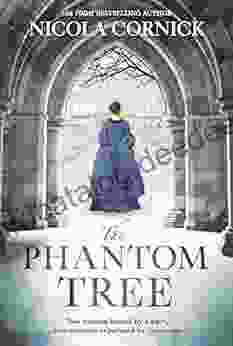

The Phantom Tree: A Novel New Timeslip that Transcends...
Prepare to be swept...
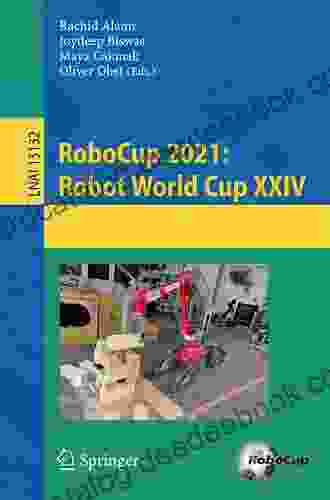

Robot World Cup XXI: Lecture Notes in Computer Science...
The 21st Robot World Cup...
4.8 out of 5
Language | : | English |
File size | : | 7598 KB |
Text-to-Speech | : | Enabled |
Enhanced typesetting | : | Enabled |
Word Wise | : | Enabled |
Print length | : | 347 pages |
Screen Reader | : | Supported |
Paperback | : | 104 pages |
Reading age | : | 9 - 12 years |
Grade level | : | 4 - 6 |
Item Weight | : | 4 ounces |
Dimensions | : | 5 x 0.24 x 8 inches |