Biomedical Image Registration Domain Generalisation and Out of Distribution

Biomedical image registration is a fundamental task in medical imaging. It involves aligning two or more images of the same anatomy, acquired at different times or from different modalities, to enable accurate analysis and diagnosis. However, biomedical image registration is a challenging task due to the large variations in image appearance, shape, and size. These variations can be caused by different factors, such as patient motion, organ deformation, and changes in imaging parameters.
Domain generalisation and out of distribution (OOD) problems further complicate the task of biomedical image registration. Domain generalisation refers to the problem of training a model that can perform well on data from different domains, even if the model has not been trained on data from these domains. OOD data refers to data that is significantly different from the data that the model was trained on. OOD data can pose a challenge to models, as they may not be able to generalise well to such data.
In this article, we provide an overview of recent advances in biomedical image registration that address the challenges of domain generalisation and OOD data. We discuss different approaches to domain generalisation, including unsupervised domain adaptation, adversarial learning, and meta-learning. We also discuss methods for detecting and handling OOD data. Finally, we provide an outlook on future directions for research in this area.
4.6 out of 5
Language | : | English |
File size | : | 26860 KB |
Text-to-Speech | : | Enabled |
Screen Reader | : | Supported |
Enhanced typesetting | : | Enabled |
Print length | : | 346 pages |
Domain generalisation is a challenging problem in machine learning. The goal of domain generalisation is to train a model that can perform well on data from different domains, even if the model has not been trained on data from these domains. This is a difficult problem, as data from different domains can have different distributions, making it difficult for a model to generalise well to all of them.
There are a number of different approaches to domain generalisation, including unsupervised domain adaptation, adversarial learning, and meta-learning.
Unsupervised domain adaptation is a method of domain generalisation that does not require any labelled data from the target domain. Instead, unsupervised domain adaptation methods use unlabelled data from the target domain to learn a mapping between the source and target domains. This mapping can then be used to transform data from the source domain into the target domain, making it easier for a model to generalise to the target domain.
Adversarial learning is a method of domain generalisation that uses a generative adversarial network (GAN) to learn a mapping between the source and target domains. A GAN consists of two networks: a generator network and a discriminator network. The generator network learns to generate data that is similar to the data from the target domain, while the discriminator network learns to distinguish between data from the source and target domains. By training the generator and discriminator networks together, the generator network learns to generate data that is indistinguishable from data from the target domain, which can then be used to train a model that can generalise well to the target domain.
Meta-learning is a method of domain generalisation that learns to learn quickly from a small amount of data. Meta-learning algorithms are trained on a set of tasks, and then they can be used to quickly learn new tasks with only a few examples. This makes meta-learning algorithms ideal for domain generalisation, as they can quickly learn to generalise to new domains with only a small amount of data from those domains.
Out of distribution (OOD) data refers to data that is significantly different from the data that the model was trained on. OOD data can pose a challenge to models, as they may not be able to generalise well to such data.
There are a number of different methods for detecting and handling OOD data. One common method is to use a confidence threshold. A confidence threshold is a value that is used to determine whether or not a model is confident in its prediction. If the model's confidence is below the threshold, then the prediction is considered to be OOD.
Another method for detecting and handling OOD data is to use a rejection network. A rejection network is a network that is trained to identify OOD data. The rejection network can be used to filter out OOD data before it is used to train a model.
The research on biomedical image registration domain generalisation and OOD data is still in its early stages. However, there are a number of promising directions for future research.
One promising direction for future research is to develop new methods for unsupervised domain adaptation. Unsupervised domain adaptation methods do not require any labelled data from the target domain, making them ideal for
4.6 out of 5
Language | : | English |
File size | : | 26860 KB |
Text-to-Speech | : | Enabled |
Screen Reader | : | Supported |
Enhanced typesetting | : | Enabled |
Print length | : | 346 pages |
Do you want to contribute by writing guest posts on this blog?
Please contact us and send us a resume of previous articles that you have written.
Book
Chapter
Story
Reader
Library
Magazine
Glossary
Bibliography
Foreword
Preface
Synopsis
Footnote
Tome
Library card
Narrative
Biography
Autobiography
Memoir
Reference
Character
Resolution
Librarian
Catalog
Card Catalog
Borrowing
Stacks
Archives
Periodicals
Study
Lending
Reserve
Academic
Journals
Reading Room
Interlibrary
Study Group
Thesis
Awards
Book Club
Textbooks
Brenda Billings Ridgley
Deborah Meier
Reece Lumsden
James Gould
Kevin Desmond
Richard Mcculloch
Richard Godbeer
Jenny King
Linda Herrera
Aimee Bahng
Jahan Brian Ihsan
Razaq Adekunle
Aiden Thomas
Devlin Barrett
Aitor Oyanguren Uriarte
Akheel Mohammad
Roxann Read
Walter A Strauss
Trevor Fry
Stanley Laine
Light bulbAdvertise smarter! Our strategic ad space ensures maximum exposure. Reserve your spot today!
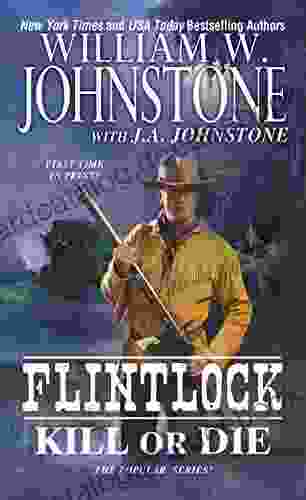

- Paul ReedFollow ·11.4k
- Marcel ProustFollow ·16k
- Hugh BellFollow ·15.9k
- Herb SimmonsFollow ·9.1k
- Calvin FisherFollow ·14.2k
- William PowellFollow ·9.3k
- Russell MitchellFollow ·9.1k
- Geoffrey BlairFollow ·5.4k
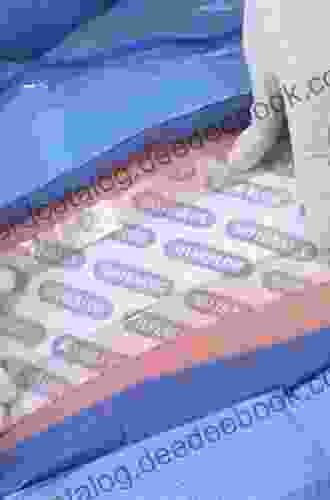

Chronic Wounds, Wound Dressings, and Wound Healing:...
Chronic wounds are a major challenge for...
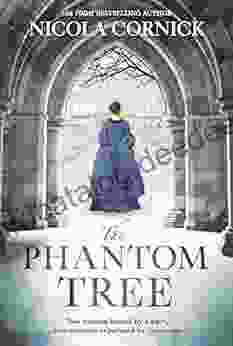

The Phantom Tree: A Novel New Timeslip that Transcends...
Prepare to be swept...
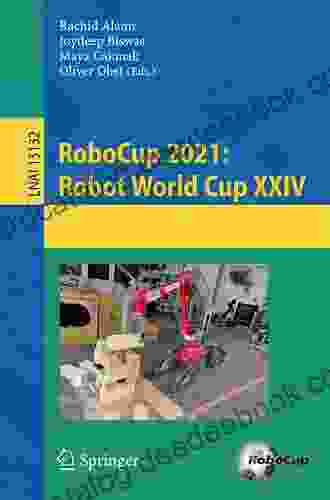

Robot World Cup XXI: Lecture Notes in Computer Science...
The 21st Robot World Cup...
4.6 out of 5
Language | : | English |
File size | : | 26860 KB |
Text-to-Speech | : | Enabled |
Screen Reader | : | Supported |
Enhanced typesetting | : | Enabled |
Print length | : | 346 pages |